Ahead of the Curve: Cross-Examining AI
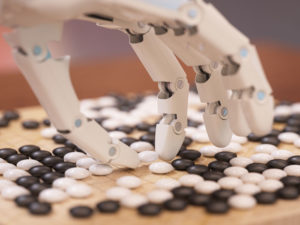
All self-respecting articles about artificial intelligence (AI) must start with AlphaGo, the AI that just trounced the human champion at the Chinese game of Go. No one thought this would happen, even though other AIs have already humbled human champions at both chess and on Jeopardy!. Such articles then move on to the specter of machines surpassing and enslaving humanity, which Elon Musk says will happen and Mark Zuckerberg says will not. The majority of writers note that the reason Zuckerberg’s forecast is (probably) more accurate than Musk’s (at least over the next few decades) is that most AI is designed for a single purpose—like playing Go, diagnosing disease, or screening job applicants. There isn’t nearly as big a market for the “make me a generic human mind, but better, that sits inside a computer until it learns how to bust out and enslave me” AI as there is for single-purpose AI to perform tasks like driving a vehicle or managing a portfolio. A single-purpose AI seems less threatening than a multipotent one, even though Robert Harris in The Fear Index suggests otherwise.
Now that I’ve got that out of the way, let’s consider an issue that already exists with AI today, even the single-purpose variety, that is bound to become even more serious in the immediate future. What happens when what seems to be perfectly sound AI starts spitting out results that are, well, weird? And when you try to find out why, you can’t?
We know AI can make mistakes. A couple of years ago, a fairly primitive AI used to assist admissions screening at St. George’s Hospital Medical School was found to be riddled with racial and gender bias. This happened not because its creators consciously intended to favor white males, but because the past data relied on to identify good candidates came from a less enlightened period.
This unfortunate episode had a happy ending. Humans spotted the problem, they figured out what was causing it, and they fixed it. But now there is a situation involving “Compas,” an AI used in a number of courts to assist in criminal sentencing. Compas relies on mountains of past data to make informed predictions about a particular convict’s future behavior under various sentencing scenarios. This is exactly what judges do, seat of the pants. There is every reason to believe that a well-designed AI with access to millions of case histories can do this job as well or better than even an experienced judge, who’s had a few thousand past cases at most and only remembers a tiny fraction of them in any detail.
So what happens when you get convicted of committing a crime, Compas recommends a six-year sentence, the judge says, “Six years it is,” and you have the temerity to ask, “Um, how did you come up with six years? I was expecting two. What was the reasoning process?”
What happens is that the firm that owns Compas responds, “Sorry, bud. Our algorithm is proprietary information. We’re not going to tell you. See you in six years.”
Is Compas racially biased? Is it gender biased? Is it sometimes just stupid? If I were the one doing the six years, I would want to explore questions like that. But, according to the courts, I can’t. The prisoner who sought the chance to discover how Compas reached its conclusion lost in court earlier this year.
This outcome seems egregious, but it’s still in the realm of things that can be fixed. Court decisions do get reversed from time to time, and this one seems like a prime candidate for a short lifespan.
Racial or other bias is one problem area, but there are others as well. Like my favorite one: money. Suppose you have a theoretically neutral AI manage certain tasks for you, like arranging travel plans, or handling your grocery shopping. How exactly do you know it’s neutral, and hasn’t been nudged toward a particular outcome in the same way that Google search results put paid advertisers at the top? Google is at least open about what it does – how do we know a black box AI, which may or may not have been hacked, will be looking out only for you?
Last week there was a story on CNN about what appears to be a massive over-prescription of a drug called Nuedexta. This drug is apparently effective at controlling a condition affecting a tiny number of elderly people, but has had sales skyrocket because of a combination of aggressive marketing and jaw-dropping ignorance (or worse) among buyers. There’s more to the story than that, but here’s my question: Suppose an AI doctor starts prescribing puzzling quantities of Drug X. This AI has a good reputation, and has helped a lot of people. But no one can figure out why it’s prescribing so much Drug X. Was it hacked? Is Drug X really that good? Does it have a positive side effect no one else understands? Does it have negative side effects that result in greater usage of the AI, which somehow makes the AI “happy?” I don’t think many readers will disagree that we will really, really need the AI to tell us, in a testable way, why it is prescribing so much Drug X.
This turns out to be far more difficult than it sounds. The earliest versions of AI were rule-driven—essentially, a vast tree of “if… then …” branches. If the applicant’s MCAT score is below a certain level, check her GPA; if that’s below a certain level, assign her to the rejection pile. The tree can be enormous, but with a little patience you can trace how it reaches any particular conclusion. The problem is, much of life is too complicated to lend itself to this kind of approach, and it is not how the brain itself normally works. Instead, both the brain and the more recent AI programs rely on “neural networks,” in which hundreds of “layers” of neurons approach multiple parts of a task simultaneously. Reconstructing how a neural network reached a conclusion, we are told, falls somewhere between “extremely difficult” and “impossible.” For example, a neural network AI called “Deep Patient” has developed an uncanny knack for predicting the onset of schizophrenia, more accurately than any human doctor. How? No one knows—it just does.
Then there’s the fact that some of the best AIs are never given a set of rules by humans in the first place, but are simply given an assignment and told to attack it by trial and error, in a technique called “backpropagation,” that bears some resemblance to Darwinian natural selection. AlphaGo is an excellent example. Its designers basically said, “Here are the rules of the game. Winning it is your goal. Now you go play millions of games against yourself, and come up with the best possible strategies for reaching that goal.” It did, and can now reach that goal better than any human being can. But if you ask its designers why it made a particular move at a critical moment, all you get is a shrug. They never taught AlphaGo what to do—and they never built in any mechanism for it to explain its reasoning.
For a board game, this deficiency is trivial. For an AI medical professional, or investment manager, or vehicle operator, or hiring officer, or military drone, it’s not trivial at all. The prospect of living in a world where it’s not possible to get reliable answers about why powerful AIs do what they do is terrifying.
In fairness, we humans are not always marvelous at answering, “Why’d you do that?” questions either. Sometimes, we even lie. But members of our species have thousands of years of collective experience in dealing with each other on matters like this, and we muddle through at an acceptable level of uncertainty. We don’t have any comparable experience of asking a powerful AI “Why?” and getting “?” in response.
So, you say, there ought to be a law. In fact there already is one, at least in the European Union, scheduled to take effect next May. As difficult as it may be get a backpropagating neural network to record its reasoning process, it can’t be impossible. But I can already hear the tech industry complaints. It’s more expensive. It’s slower—a real problem for AI in applications like self-driving vehicles, where speed of decision making is rather important. It will delay the date for bringing products to market—a death sentence in this business. It’s unreliable anyway—how do you know it’s telling the truth?
Worse yet: What’s to stop AI developers from ignoring whatever laws one country adopts and selling their products from Ukraine, or Venezuela, or Fiji? Law, and the lawyers who come with them, are not the ultimate answer.
Two AIs, both do the same thing, both have similar quality ratings. The only difference is that one is much slower and much more expensive than the other, because thanks to some bureaucrat it painstakingly records all nine trillion steps in its decision making process. Which would you buy?
This flippant question is actually the ultimate answer. We humans need to be willing to pay more [I hate saying that] for the AI that can explain itself. That’s our only strategy that has a chance of success. We need to be willing to pay attention to humans on our side who say, “Yeah, AI X is good at what it does. But so is AI Y. And AI Y does a decent job (as far as we can tell) of explaining why it does what it does.”
We need to buy AI Y. We need to boycott anyone who uses AI X. We need to frighten the future developers and financial backers of AI X into believing we are going to do this, so that it never gets developed in the first place.
Even before we get to this stark X vs. Y choice, we humans need to be aware of the explainability issue. We need to demand that knowledgeable fellow humans scrutinize AIs that are smarter than we are, at least in their one trick pony fields, and conclude satisfactorily that we can figure out why they do what they do, at least when we want to. And raise bloody hell when they don’t.