Ahead of the Curve: AI vs. Bias
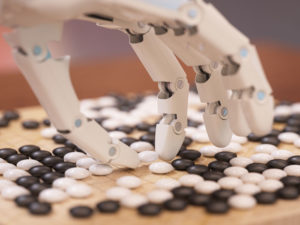
Every now and then an idea comes along that’s so elegant, so obvious (at least in hindsight), that I want to smack my forehead and exclaim, “Why didn’t I think of that? I could have been rich!” Take packaged salads, for example. The technology to clean and chop up salad ingredients and pack them in convenient meal-sized plastic bags or containers, making life a tad easier for those of us too busy or too lazy to do it ourselves, has existed for the past eighty years. But no one actually thought to do it until the 1990s. And that person wasn’t me.
My latest forehead slap involves the relationship between artificial intelligence (AI) and discrimination—on racial, gender, sexual preference, or other lines. Much has been written, including by me, about the serious flaws in what are supposedly “neutral” AI tools used to aid decisions for sentencing convicts, hiring employees, and so on. It turns out that AI is only as good as the data it’s fed. If that data is biased, the AI will be biased too.
The good news here is that many AI engineers are painfully aware of the problems and are working diligently to fix them. Not just because such bias is morally wrong, but because it’s stupid, and produces suboptimal results.
Engineers working to fix software flaws is all well and good, but not breathtaking. That distinction I will reserve for the simple idea of a professor who has made a career documenting bias in the American justice system. Succinctly: instead of just having humans grouse about AI bias, why not create an AI to identify human bias? There’s certainly plenty of it to be found.
Daniel Chen, now at the University of Toulouse, has spent years analyzing mountains of data about the US judicial system to locate evidence of bias. He’s found lots of it, some surely unconscious. For example, consider the administrative law judges who handle immigration asylum cases. Chen has found widespread incidence of what’s called the “gambler’s fallacy”—i.e., if the ball in the roulette wheel hits black three times in a row, surely there’s a greater chance it will hit red next time, right? Wrong—the chance is 47.4 percent, as it’s always been. But what happens when a judge grants three asylum applications in a row? What happens is, you don’t want your application to be next in line, because the judge subconsciously feels he or she is being overgenerous, and it’s time to get back to an even keel. Chen’s data proves this happens, and not just with immigration judges. It happens with loan officers, who are more likely to lean back the other way after approving or disapproving several loan applications in a row. It even happens with baseball umpires, calling balls and strikes.
If you ever get convicted of a crime, try to have your lawyer arrange for you to be sentenced on your birthday. Chen has found such sentences to be lighter than they are on the other 364 days of the year – by as much as 15 percent in one jurisdiction.
Chen has also uncovered more insidious forms of bias. Turning back to immigration judges, he finds clear evidence that many are biased either for or against asylum applicants based on their country of origin. He goes so far as to claim that if you tell him the name of the judge and the country of origin of the applicant, he can predict the case outcome with 80 percent accuracy—without knowing any other facts. That is disheartening, to say the least.
I’ve read some of Chen’s papers, and I will admit to not understanding the jargon well enough to decide whether his techniques constitute full-blown AI as we’ve come to know it, or just a glorified regression analysis, which I used to dimly understand. Chen understands, though. He credits “machine learning” with the asylum judge bias discovery, and he’s anxious to ratchet up the machine second-guessing of decision-makers to the next level.
“I’d love to have a large dataset on the history of the judge’s decisions and all of the potential contextual extraneous factors,” he says. “Then you could analyze the data and see what factors, relevant and not relevant, might have affected the judge’s decision. A big dataset can help us say that in these certain situations, the judge is more likely to be influenced in a given direction.”
The goal is not to prove that judges are bigots and the system is a sham, but to help them get better. Lots of people, no doubt including me, are influenced by improper factors, no matter how hard we try not to be. If someone comes up to me and says, “Your decision making sucks—it’s riddled with prejudice,” I’m likely to resist (and probably rudely). But if someone says, “Here’s data to prove you’re not as fair as you’d like to be, and here are the particular pitfalls you need to watch for,” then I would take that constructive criticism seriously.
That’s exactly what Chen is calling for in his latest paper, “Machine Learning and the Rule of Law.” As he puts it, “The advent of machine learning tools and their integration with legal data offers a mechanism to detect in real time, and thereby remedy judicial behavior that undermines the rule of law.” He then sets out the framework for future algorithms to do exactly that, in the hope that: “Simply alerting judges to the fact that their behavior is highly predictable in ways that may indicate unfairness may be sufficient to change their behavior.” If that doesn’t work, public shaming might help, or perhaps the threat of action from superiors.
Let’s hope that Chen succeeds in generating plenty more activity in the direction he is pushing. Not just for judges, which have been his principal focus, but for decision-makers in corporate hiring, school admissions, policing, and elsewhere—even sports officiating. It’s not hard to envision a time when the public demands that institutions employ bias-checking AI, if it can be made to work, and if the basis for its conclusions is transparent. What’s the good reason not to use it?